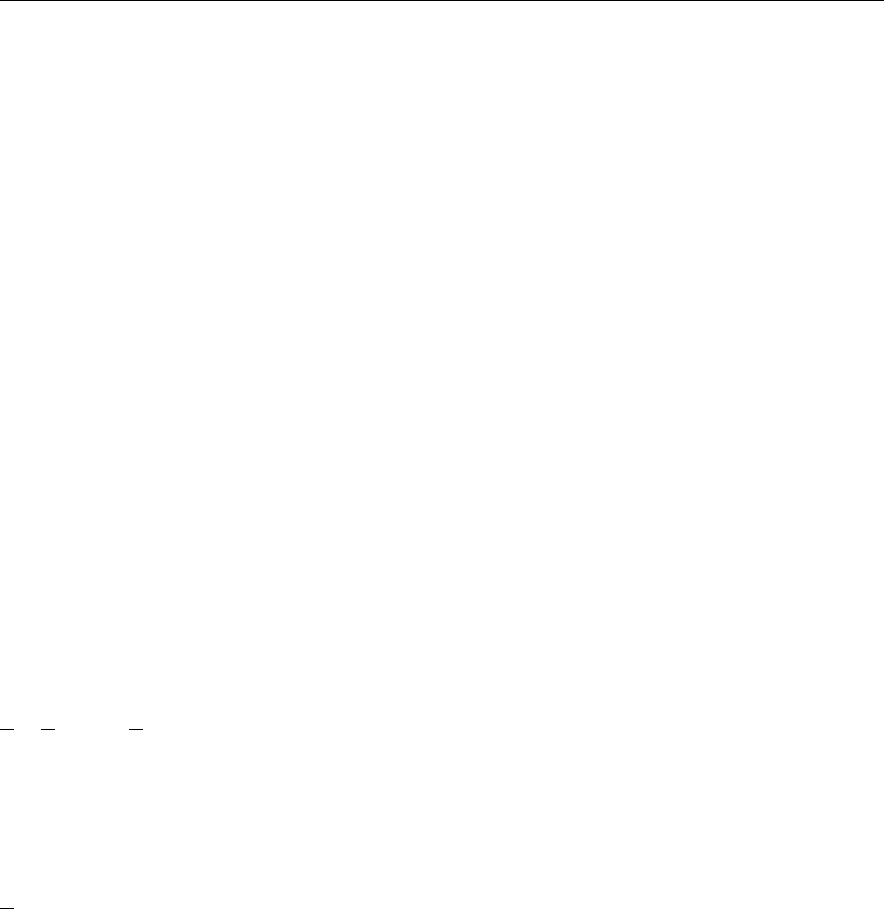
Gauthier, Cline, Alfred Lehar, and Moez Souissi, “Macroprudential capital requirements and
systemic risk,” Journal of Financial Intermediation, 2012, 21 (4), 594 – 618.
Gerardi, Kristopher, Andreas Lehnert, Shane Sherlund, and Paul Willen, “Making Sense
of the Subprime Crisis,” Brookings Papers on Economic Activity, 2008, 39 (2 (Fall)), 69–159.
Gerlach, Stefan and Wensheng Peng, “Bank lending and property prices in Hong Kong,” Journal
of Banking and Finance, 2005, 29 (2), 461–481.
Goldberg, Lawrence and Charles A. Capone, “A Dynamic Double-Trigger Model of Multifamily
Mortgage Default,” Real Estate Economics, 2002, 30 (1), 85–113.
Grovenstein, Robert A., John P. Harding, C.F. Sirmans, Sansanee Thebpanya, and Geof-
frey K. Turnbull, “Commercial mortgage underwriting: How well do lenders manage the risks?,”
Journal of Housing Economics, 2005, 14 (4), 355–383.
Harrell, F.E., Regression Modeling Strategies: With Applications to Linear Models, Logistic Regres-
sion, and Survival Analysis Springer Series in Statistics, Springer New York, 2001.
Haughwout, Andrew, Richard Peach, and Joseph Tracy, “Juvenile delinquent mortgages: Bad
credit or bad economy?,” Journal of Urban Economics, 2008, 64 (2), 246 – 257.
Igan, Deniz and Heedon Kang, “Do Loan-To-Value and Debt-To-Income Limits Work? Evidence
From Korea,” IMF Working Papers 11/297, International Monetary Fund 2011.
IMF, “Key Aspects of Macro-prudential Policy,” Background Paper, IMF 2013.
Jacome, Luis and Srobona Mitra, “Experience with LTV and DTI Limits - Lessons Through a
Magnifying Glass,” IMF Working Paper, IMF 2015.
Jiang, Wei, Ashlyn Aiko Nelson, and Edward Vytlacil, “Securitization and Loan Performance:
Ex Ante and Ex Post Relations in the Mortgage Market,” Review of Financial Studies, 2013.
, , and , “Liar’s Loan? Effects of Origination Channel and Information Falsification on
Mortgage Delinquency,” The Review of Economics and Statistics, 2014, 96 (1), 1–18.
Kashyap, Anil K, Richard Berner, and Charles A E Goodhart, “The Macroprudential
Toolkit,” IMF Economic Review, 2011, 59 (2), 145–161.
Kelly, Robert and Terence OMalley, “The good, the bad and the impaired: A credit risk model
of the Irish mortgage market,” Journal of Financial Stability, 2016, 22, 1 – 9.
, Terry O’Malley, and Conor O’Toole, “Designing Macro-prudential Policy in Mortgage Lend-
ing: Do First Time Buyers Default Less?,” Research Technical Papers 02/RT/15, Central Bank of
Ireland 2015.
Kennedy, Gerard and Tara McIndoe Calder, “The Irish Mortgage Market: Stylised Facts,
Negative Equity and Arrears,” Research Technical Papers 12/RT/11, Central Bank of Ireland 2011.
Lydon, Reamonn and Yvonne McCarthy, “What Lies Beneath? Understanding Recent Trends
in Irish Mortgage Arrears,” The Economic and Social Review, 2013, 44 (1), 117–150.
Marrie, Ruth Ann, Neal V. Dawson, and Allan Garland, “Quantile regression and restricted
cubic splines are useful for exploring relationships between continuous variables,” Journal of Clinical
Epidemiology, 2009, 62 (5), 511 – 517.e1.
McCann, Fergal, “Modelling default transitions in the UK mortgage market,” Research Technical
Papers 18/RT/14, Central Bank of Ireland 2014.
Nabar, Malhar and Ashvin Ahuja, “Safeguarding Banks and Containing Property Booms; Cross-
Country Evidenceon Macroprudential Policies and Lessons From Hong Kong SAR,” IMF Working
Papers 11/284, International Monetary Fund 2011.
25